Progress in Cancer Diagnostics: The Pathomics Initiative by AroraLab
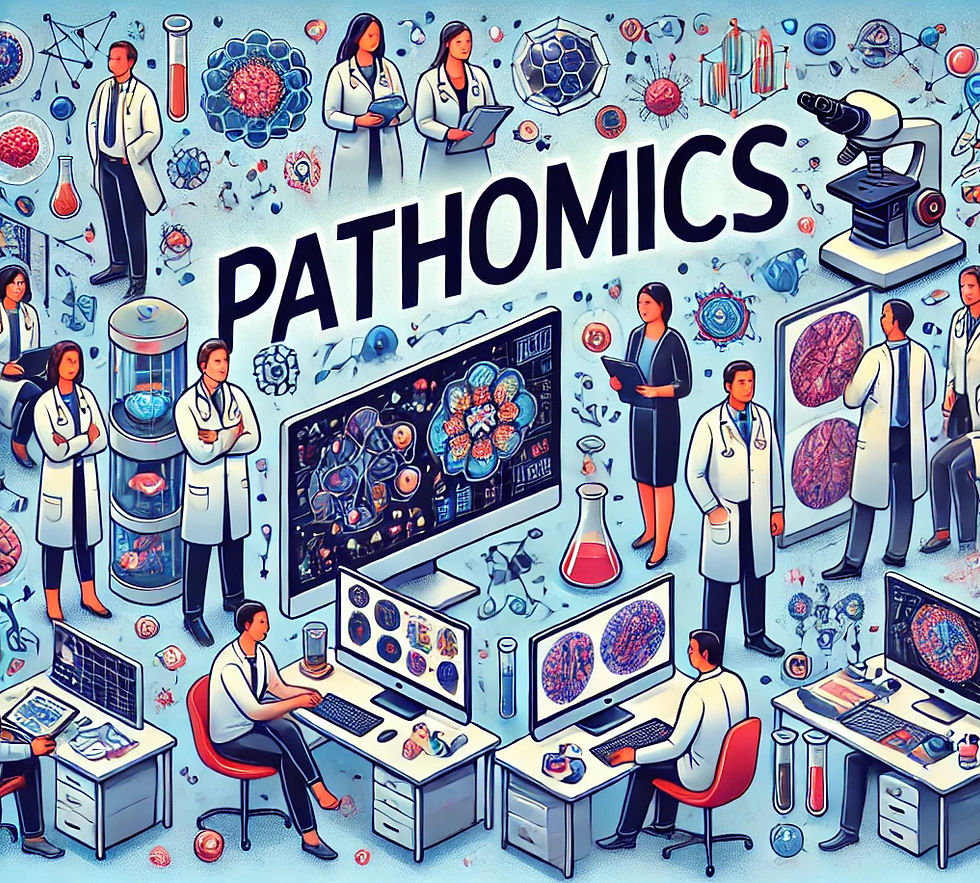
Early Detection and Accurate Prognosis: The Need for Innovation
Early detection and accurate prognosis assessment are significant challenges in the diagnosis and treatment of cancer. The lack of these can increase the risk of death and overall disease risk, as well as the cost of treatment. While clinical testing strategies are effective, recent progress in machine learning has shown potential for developing pipelines that automate standardized and objective assessments, thus reducing time, human capital, and other resources.
The Role of AI in Pathology
Current digital imaging analysis tools use Artificial Intelligence (AI) tools to identify cancer areas in digital pathology and distinguish them from non-cancerous regions. However, these tools have certain limitations that restrict their clinical use. For instance, they rely only on histological architecture to grade cancers, have minimal capability to distinguish between proximal cancer areas, and cannot predict cancer progression/regression. One of the primary reasons for these limitations is that AI models rely on large amounts of clinical data for training, which is often biased, lacks diversity, and is not readily available.
The Pathomics Initiative: Overcoming Limitations
To address these issues, the Arora Lab has initiated the Pathomics project. This project integrates advanced generative adversarial network (GAN) models, in-house quantification models, and multi-Omic tumor profiling data to create high-quality synthetic images of different cancer grades. These images account for the granularity of each tumor grade, potentially substituting real histology data for training convolutional neural network (CNN) models and evaluating the improvement in their grading efficiency.
Preliminary Research and Findings
In our preliminary research, we processed multi-omics data from patients with prostate adenocarcinoma. We then evaluated various GAN models to generate synthetic data of cancer histology and settled on the most efficient one. Additionally, we created spatial quantification modules to analyze the images. Using these synthetic images, we trained a CNN model and compared its performance with a model trained solely on real images. The results showed a significant improvement in grading efficiency when synthetic images were included in the training data.
Expanding Our Vision
While our initial pipelines have been developed using prostate cancer data, our vision is to extend this approach to other cancer types. The Pathomics initiative aims to enhance early detection and prognosis of various cancers through improved AI sensitivity to early cancer signs, comprehensive omics profiling, and predictive analytics for timely intervention. Overall, it aims to reduce healthcare costs by streamlining cancer diagnosis.
Call to Action
Achieving these goals requires the collective expertise of professionals from diverse backgrounds. We invite experts in pathology, molecular endocrinology, oncology, industrial and biomedical engineering, machine learning, data science, and statistics to join us in this transformative journey. Together, we can push the boundaries of AI in healthcare and significantly improve patient outcomes in cancer diagnostics.
If you have expertise and interest in this field, please reach out to Dr. Himanshu Arora. Join us in the Pathomics initiative and be part of the revolution in digital pathology and AI-driven healthcare innovation!
Comentários