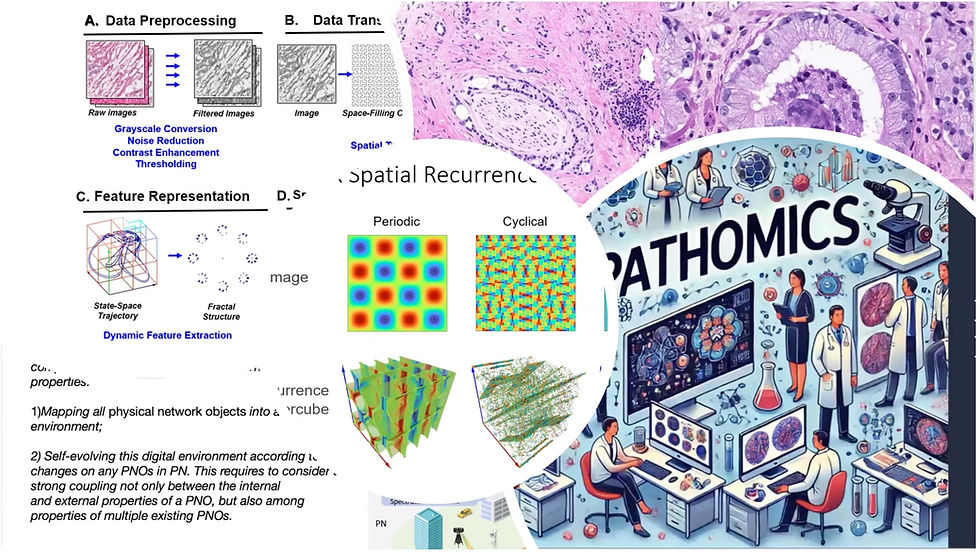
The 2nd Pathomics Initiative meeting brought together leading experts in pathology, genomics, AI, and machine learning to discuss recent advancements and ongoing efforts to revolutionize cancer diagnostics. The session was rich with insights into the latest updates on data acquisition, quantification models, genomics, and innovative simulation frameworks. Below are the key highlights from the meeting:
Recent PLCO Data Acquisition: Expanding the Data Pool
One of the most significant updates discussed in the meeting was related to the recent Prostate, Lung, Colorectal, and Ovarian (PLCO) Cancer Screening Trial data acquisition. This dataset is invaluable for improving early cancer detection, especially for prostate cancer, by providing large-scale, diverse, and longitudinal data. The expanded dataset will enable more robust training of AI models used for cancer grading, particularly for identifying subtle cancerous changes in histology images. By incorporating this newly acquired PLCO data, the Pathomics Initiative is positioned to enhance model accuracy, reduce bias, and diversify the dataset pool, addressing key challenges faced by earlier AI tools in digital pathology.
Granular Nuances in Gleason Scoring: Insights from Pathologists
Gleason scoring remains one of the most critical components in the pathological assessment of prostate cancer. However, a central topic of discussion during the meeting was the complexity of accurately determining Gleason scores, particularly in cases where the cancerous architecture is ambiguous. Pathologists highlighted several granular nuances that must be taken into account when assessing tissue samples:
Micro-level structural variances: Subtle differences in cell arrangements and glandular structure can drastically affect the score.
Heterogeneity in tissue samples: Tumor heterogeneity within the same sample presents challenges for consistent grading.
Observer variability: Inter-observer variability continues to be a challenge, emphasizing the need for standardized AI-based quantification models.
The meeting underscored the importance of addressing these nuances, as AI models should be trained not only on general patterns but also on subtle changes that pathologists rely on when making crucial diagnostic decisions.
Updates on Quantification Models: Assimilating Pathological Insights
The meeting featured significant advancements in the development of quantification models, particularly those designed to replicate how pathologists interpret histological features. The updated models focus on assimilating key features identified by pathologists when making calls regarding cancer grade and type, such as glandular structure, cellular density, and tumor heterogeneity.
These models now integrate advanced spatial analysis techniques that break down histological images into more granular components, allowing for a more nuanced assessment. Additionally, these models offer:
Improved sensitivity to early-stage cancer markers.
Higher accuracy in distinguishing between similar cancer types.
Automation of key pathology processes, reducing human error.
This fusion of expert pathological insight with advanced AI models represents a major leap toward creating diagnostic tools that can match or exceed human pathologist performance, ensuring more consistent, accurate results.
Genomics Normalization: Strengthening the Framework
Another core topic discussed at the meeting was the recent progress in genomics normalization techniques. Genomics data, when combined with histology images, provides a multi-dimensional view of cancer that greatly enhances diagnostic accuracy. However, inconsistencies and noise in genomic datasets have historically posed challenges for AI model training.
The new normalization techniques discussed aim to correct for:
Batch effects, ensuring that data from different sources are comparable.
Noise reduction, filtering out irrelevant signals that may skew AI predictions.
Cross-platform compatibility, enabling seamless integration with histology data from diverse platforms.
The integration of normalized genomics data will allow AI models to incorporate genetic information more reliably into their predictions, improving cancer prognosis and guiding personalized treatment decisions.
Digital Twin Modeling: Advancing Simulation Capabilities
Digital twin modeling is an innovative approach that is set to significantly advance the Pathomics Initiative's cancer research framework. During the meeting, it was revealed that the team has made significant strides in using digital twins for simulation purposes. A digital twin is a virtual replica of a biological entity—in this case, a cancer patient—that can simulate various disease progressions and treatment responses.
The application of digital twins in cancer diagnostics and treatment modeling offers several advantages:
Personalized simulations: Tailoring treatment plans based on individual patient data, including genomics, pathology images, and clinical history.
Predictive analytics: Running simulations to forecast disease progression or regression under different treatment scenarios.
Real-time adaptability: Adjusting the model as new data becomes available, offering dynamic and more accurate predictions.
By incorporating digital twin technology, the Pathomics Initiative aims to create a framework that not only assists in early detection but also allows clinicians to simulate potential treatment outcomes, leading to more personalized and effective interventions.
Call to Action: Join the Pathomics Initiative
As we continue to push the frontiers of AI-driven cancer diagnostics, we invite experts, researchers, and organizations from diverse fields to collaborate with us on the Pathomics Initiative. Whether you're a pathologist, data scientist, oncologist, or molecular biologist, your expertise can contribute to the transformative innovations being developed in cancer detection and treatment.
The progress shared at the 2nd Pathomics Initiative meeting marks just the beginning. There are countless opportunities to make a significant impact on how we understand, diagnose, and treat cancer. By joining forces, we can create smarter, more personalized diagnostic tools that improve patient outcomes and revolutionize oncology care.
If you're interested in collaborating, attending future meetings, or contributing your research, please reach out to us. Together, we can build the next generation of diagnostic technology and make early cancer detection and accurate prognosis a reality.
Contact us at the Pathomics Initiative to be a part of this exciting journey towards a future of smarter, more efficient healthcare.
Yorumlar